INFFC: An iterative class noise filter based on the fusion of classifiers with noise sensitivity control
This Website contains complementary material to the paper:
José A. Sáez, Mikel Galar, Julián Luengo, Francisco Herrera, INFFC: An iterative class noise filter based on the fusion of classifiers with noise sensitivity control. Information Fusion, Information Fusion 27 (2016) 505-636. doi: 10.1016/j.inffus.2015.04.002.
The web is organized according to the following summary:
Abstract
In classification, noise may deteriorate the system performance and increase the complexity of the models built. In order to mitigate its consequences, several approaches have been proposed in the literature. Among them, noise filtering, which removes noisy examples from the training data, is one of the most used techniques. This paper proposes a new noise filtering method that combines several filtering strategies in order to increase the accuracy of the classification algorithms used after the filtering process. The filtering is based on the fusion of the predictions of several classifiers used to detect the presence of noise. We translate the idea behind multiple classifier systems, where the information gathered from different models is combined, to noise filtering. In this way, we consider the combination of classifiers instead of using only one to detect noise. Additionally, the proposed method follows an iterative noise filtering scheme that allows us to avoid the usage of detected noisy examples in each new iteration of the filtering process. Finally, we introduce a noisy score to control the filtering sensitivity, in such a way that the amount of noisy examples removed in each iteration can be adapted to the necessities of the practitioner. The first two strategies (use of multiple classifiers and iterative filtering) are used to improve the filtering accuracy, whereas the last one (the noisy score) controls the level of conservation of the filter removing potentially noisy examples. The validity of the proposed method is studied in an exhaustive experimental study. We compare the new filtering method against several state-of-the-art methods to deal with datasets with class noise and study their efficacy in three classifiers with different sensitivity to noise.
Datasets
The experimentation is based on 25 datasets from the KEEL-dataset repository. They are described in the following table, where #EX refers to the number of examples, #AT to the number of attributes and #CL to the number of classes. Examples containing missing values are removed from the datasets before their usage.
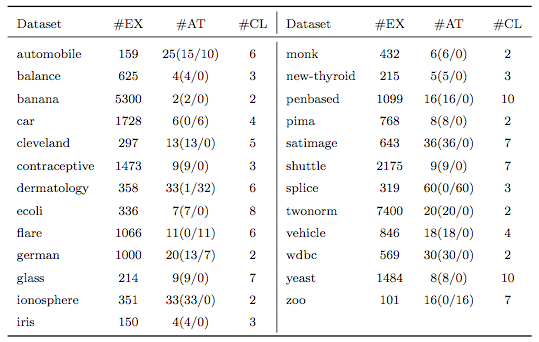
You can also download all these datasets by clicking here:
Performance results
In the following table you can download the files with the results of each method considered and the analysis of the examples affected by our proposal.
Accuracy | Examples |
---|---|
![]() |
![]() |