Genetic learning of accurate and compact fuzzy rule based systems based on the 2-tuples linguistic representation 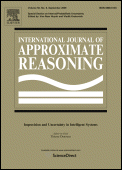 | R. Alcalá, J. Alcalá-Fdez, F. Herrera, J. Otero, Genetic Learning of Accurate and Compact Fuzzy Rule Based Systems Based on the 2-Tuples Linguistic Representation. International Journal of Approximate Reasoning 44:1 (2007) 45-64, doi:10.1016/j.ijar.2006.02.007. |   |
Abstract: One of the problems that focus the research in the linguistic fuzzy modeling area is the trade-off between interpretability and accuracy. To deal with this problem, different approaches can be found in the literature. Recently, a new linguistic rule representation model was presented to perform a genetic lateral tuning of membership functions. It is based on the linguistic 2-tuples representation that allows the lateral displacement of a label considering an unique parameter. This way to work involves a reduction of the search space that eases the derivation of optimal models and therefore, improves the mentioned trade-off.
Based on the 2-tuples rule representation, this work proposes a new method to obtain linguistic fuzzy systems by means of an evolutionary learning of the data base a priori (number of labels and lateral displacements) and a simple rule generation method to quickly learn the associated rule base. Since this rule generation method is run from each data base definition generated by the evolutionary algorithm, its selection is an important aspect. In this work, we also propose two new ad hoc data-driven rule generation methods, analyzing the influence of them and other rule generation methods in the proposed learning approach. The developed algorithms will be tested considering two different real-world problems.
Summary: 1. Introduction
2. Rule representation based on the linguistic 2-tuples
3. Evolutionary algorithm for learning of the knowledge base
4. Two new ad hoc data-driven rule generation methods and their integration in the evolutionary learning of the DB a priori
5. Experimental study
6. Conclusions
Experimental study: - Algorithms analyzed: WM, MM, COR, WM+GL, Gr-MF, GA-WM, GA-COR.
- Data sets used: ZIP file
 - Regression: [5-fcv] ele-1, ele-2.
- Results obtained: ZIP file
Additional experimentation:
- Description: This second experimental study contains a comparison of the algorithm presented in the paper (GLD-WM) with other methods:
- MP - Multilayer perceptron
- SMOreg - Sequential Minimal Optimization
- WM - Wang and Mendel's algorithm
- CHV - Cordon, Herrera and Villar's algorithm
- GLD-WM - Gr. + Global lateral parameters + RB by WM
We provide the results obtained by employing 50000 and 100000 evaluations of the fitness function (both using MSE (Mean Squared Error) and MSE/2.
- Data sets used: ZIP file
 - Regression: [5-fcv] compactiv, concrete, dee, diabetes, laser, mortgage, treasury, wankara.
- Results obtained: ZIP file
|